AI Models And Their Technical Details
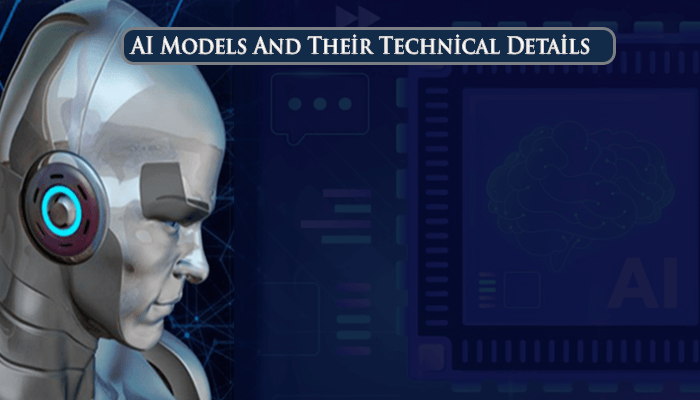
AI Models And Their Technical Details
AI models and their technical details, Let’s break down some influential AI models in research with a focus on their architecture, functionality, and key applications:
1. AlphaFold (DeepMind)
-
Model Type: Deep Learning (Transformer-based architecture)
-
Key Feature: Predicts 3D protein structures from amino acid sequences with atomic accuracy.
-
Technical Details:
-
Uses a modified Transformer model with attention mechanisms tailored for protein structure prediction.
-
Incorporates multiple sequence alignments (MSAs) to compare evolutionary data across species.
-
-
Applications: Drug design, enzyme engineering, and genetic disorder research.
2. BioGPT (Microsoft)
-
Model Type: Transformer-based Language Model
-
Key Feature: Specializes in biomedical text generation, information extraction, and knowledge discovery.
-
Technical Details:
-
Fine-tuned on vast datasets of biomedical literature (e.g., PubMed).
-
Leverages domain-specific tokenization to improve comprehension of medical jargon.
-
-
Applications: Literature review automation, clinical trial analysis, and research paper drafting.
3. ChemBERTa (Meta AI)
-
Model Type: Transformer-based model for molecular structure prediction
-
Key Feature: Encodes molecular data using the SMILES notation (a text-based representation of molecules).
-
Technical Details:
-
Trained with unsupervised learning on large chemical libraries.
-
Predicts molecular properties, toxicity, and reactivity.
-
-
Applications: Drug discovery, materials innovation, and chemical synthesis.
4. Graph Neural Networks (GNNs)
-
Model Type: Deep Learning framework for data represented as graphs
-
Key Feature: Excels at analyzing relationships and interactions between complex data points.
-
Technical Details:
-
GNNs propagate information across connected nodes, making them ideal for molecular structure analysis.
-
Key variants like Graph Convolutional Networks (GCNs) and Graph Attention Networks (GATs) are widely used.
-
-
Applications: Predicting protein-protein interactions, material stability, and social network behaviors.
5. NVIDIA’s MONAI (Medical Open Network for AI)
-
Model Type: Open-source AI framework for healthcare imaging
-
Key Feature: Provides pre-trained models optimized for medical imaging tasks.
-
Technical Details:
-
Uses state-of-the-art architectures like U-Net and Swin Transformer for segmentation and diagnosis.
-
Designed to support radiology, pathology, and surgical planning.
-
-
Applications: Cancer detection, organ segmentation, and 3D medical imaging.
Emerging Innovations
-
Diffusion Models: Applied in drug discovery to simulate molecular interactions and predict compound behaviors.
-
Reinforcement Learning (RL): Used in automated lab experiments to optimize research strategies.