Technical Breakdown Of Key Architectures
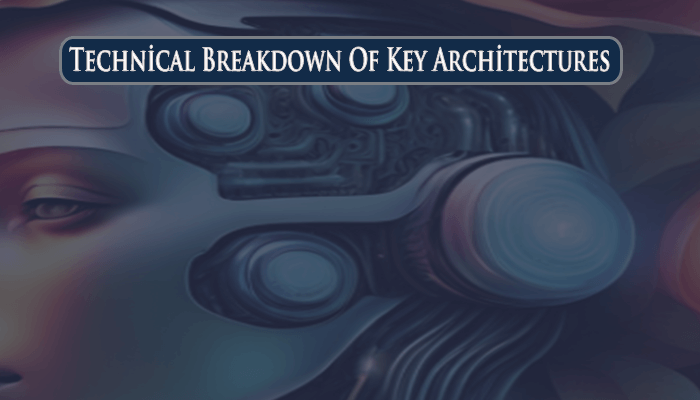
Technical Breakdown Of Key Architectures
Technical breakdown of key architectures, Let’s dive into the technical breakdown of key architectures commonly used in AI research:
1. Transformer Architecture (Core of Models like AlphaFold, BioGPT, ChemBERTa)
The Transformer model revolutionized deep learning by introducing self-attention mechanisms for improved sequence processing.
Key Components:
🔹 Input Embedding:
-
Converts data (e.g., text, protein sequences) into dense vectors that represent meaningful features.
🔹 Positional Encoding:
-
Since Transformers lack recurrence (like RNNs), they use sinusoidal functions to encode positional information.
🔹 Multi-Head Self-Attention (MHSA):
-
Enables the model to focus on multiple aspects of the input sequence simultaneously.
-
Each “head” processes information from different parts of the sequence.
Formula:
Attention(Q,K,V)=softmax(QKTdk)V\text{Attention}(Q, K, V) = \text{softmax} \left( \frac{QK^T}{\sqrt{d_k}} \right) V
Where:
-
Q = Query (what we’re focusing on)
-
K = Key (reference points in the sequence)
-
V = Value (the actual data points)
-
dkd_k = Dimension of the key vector (scaling factor to stabilize gradients)
🔹 Feed-Forward Network (FFN):
-
Fully connected layers that refine the attention outputs.
🔹 Residual Connections & Layer Normalization:
-
Ensures gradient stability and faster convergence.
2. Graph Neural Networks (GNNs)
GNNs excel at processing data structured as graphs (e.g., molecules, protein interactions).
Key Concepts:
🔹 Node Features:
-
Each node represents an entity (e.g., atoms in a molecule).
🔹 Edge Features:
-
Define the relationships between nodes (e.g., chemical bonds).
🔹 Message Passing:
-
Information propagates across connected nodes.
Formula for Node Update:
hi(l+1)=σ(W⋅∑j∈N(i)hj(l))h_i^{(l+1)} = \sigma \left( W \cdot \sum_{j \in N(i)} h_j^{(l)} \right)
Where:
-
hih_i = Node feature vector
-
N(i)N(i) = Neighbor nodes
-
WW = Learnable weight matrix
-
σ\sigma = Activation function (e.g., ReLU)
3. Diffusion Models (Emerging in Drug Discovery & Materials Science)
Diffusion models generate data by reversing a noise-injection process.
Key Steps:
🔹 Forward Process:
-
Gradually adds noise to a data sample until it becomes pure noise.
🔹 Reverse Process (Denoising):
-
Uses a neural network to reconstruct the original data from the noisy version.
Mathematical Model:
pθ(x0∣xt)=N(x0;μθ(xt,t),Σθ(xt,t))p_{\theta}(x_0 | x_t) = \mathcal{N}(x_0; \mu_{\theta}(x_t, t), \Sigma_{\theta}(x_t, t))
Where:
-
x0x_0 = Original data
-
xtx_t = Noisy data at time step tt
-
μθ\mu_{\theta} = Predicted mean by the model
-
Σθ\Sigma_{\theta} = Predicted variance
4. U-Net (Used in MONAI for Medical Imaging)
U-Net is a convolutional neural network (CNN) designed for image segmentation.
Key Features:
🔹 Encoder-Decoder Structure:
-
The encoder extracts features, while the decoder reconstructs the segmented output.
🔹 Skip Connections:
-
Links between encoder and decoder layers retain detailed spatial information.
Choosing the Right Model
-
For text-heavy research: Transformer models like BioGPT excel.
-
For molecular and structural data: GNNs offer powerful insights.
-
For image analysis in medicine or materials science: U-Net and MONAI are ideal.
-
For generative data creation: Diffusion models are gaining traction.